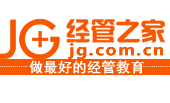
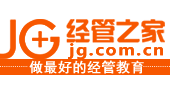
laatste wijziging: 17-11-2006
Nonlinear Time Series Models in Empirical Finance
by
Philip Hans Franses and Dick van DijkPublished by Cambridge University Press (June 2000)Outline
In this book we discuss nonlinear time series models for financial time series, which can be used for generating out-of-sample forecasts for returns and volatility. The reason for considering nonlinear models is the observation that many financial time series display typical nonlinear characteristics, as documented in the first two chapters. Important examples of those features are the occasional presence of (sequences of) aberrant observations and the possible existence of regimes within which returns and volatility display different dynamic behaviour. Through an extensive forecasting experiment (for a wide range of daily data on stock markets and exchange rates), we also demonstrate that linear time series models do not yield reliable forecasts. Of course, this does not automatically imply that nonlinear time series models would, but, as we argue in this book, it can be worth a try. As there is a host of possible nonlinear time series models, we decide to review in Chapters 3, 4 and 5, the, what we believe, currently most relevant ones and the ones that are most likely to persist as practical descriptive and forecasting devices.
In Chapter 3, we discuss several regime-switching models such as the self-exciting threshold model, the smooth transition model and the Markov switching model. In this chapter we confine the analysis to the returns on financial assets, although they can also be considered for measures of risk (or volatility) like squared or absolute returns. We consider tools for specifying, estimating, evaluating and forecasting with these models. Illustrations for several empirical series show that these models could be quite useful in practice.
In Chapter 4, we consider similar kinds of regime-switching models for unobserved volatility, which in fact amount to various extensions of the basic GARCH model. This well-known and often applied model exploits the empirical regularity that aberrant observations in financial time series appear in clusters (thereby indicating periods of high volatility), and hence that out-of-sample forecasts for volatility can be generated. The models in Chapter 4 mainly challenge the assumption in the basic GARCH model that the model parameters are constant over time and/or that positive and negative news have the same impact on subsequent volatility. Indeed, the empirical analysis in this chapter shows that a relaxation of these assumptions seems worthwhile to consider. Again, we discuss tools for specification, estimation and evaluation, and we outline how out-of-sample forecasts can be generated and evaluated.
Finally, in Chapter 5, we deal with a currently fashionable class of models, that is, with artificial neural networks. In contrast to the prevalent strategy in the empirical finance literature (which may lead people to believe that these models are merely a passing fad), we decide to `open up the black box', so to say, and to explicitly demonstrate how and why these models can be useful in practice. Indeed, the empirical applications in this chapter suggest that neural networks can be quite useful for out-of-sample forecasting and for recognizing a variety of patterns in the data.
This outline, together with the detailed table of contents can be downloaded here. Click here to order the book at CUP.
Data
The data that are used in the book consist of eight indices of major stock markets and eight exchange rates vis-à-vis the US dollar. We employ the indices of the stock markets in Amsterdam (EOE), Frankfurt (DAX), Hong Kong (Hang Seng), London (FTSE100), New York, (S&P 500), Paris (CAC40), Singapore (Singapore All Shares) and Tokyo (Nikkei). The exchange rates are the Australian dollar, British pound, Canadian dollar, German DeutschMark, Dutch guilder, French franc, Japanese yen and the Swiss franc, all expressed as number of units of the foreign currency per US dollar. The sample period for the stock indexes runs from January 6, 1986 until December 31, 1997, whereas for the exchange rates the sample covers the period from January 2, 1980 until December 1997. The original series are sampled at daily frequency. The sample periods correspond with 3127 and 4521 observations for the stock market indexes and exchange rates, respectively. We often analyze the series on a weekly basis, in which case we use observations recorded on Wednesdays. The stock market data have been obtained from Datastream, whereas the exchange rate data have been obtained from the Federal Reserve Bank of New York.
The data can be downloaded as GAUSS datasets, Eviews workfiles or Excel worksheets. All Zip-archives contain text files with the original data as well.
GAUSS programs
All computations in the book have been performed using GAUSS, version 3.2.35. Most programs and procedures can be downloaded below. There is no copyright, so feel free to use the code in your own research or teaching. There are no performance guarantees. If you use the code extensively in your research, an appropriate attribution and thanks is highly appreciated.
In writing these programs and procedures, we benefited greatly from code supplied by numerous other people. We specifically would like to mention Gerrit Draisma, Bruce Hansen, Ruud Koning, André Lucas, Stefan Lundbergh and Richard Paap.
The GAUSS programs and procedures are organized in separate Zip-archives for the different chapters which can be downloaded below. Each Zip-archive contains a readme.txt file, describing the various programs in some detail. All programs are reasonably simple and fairly well annotated internally, so you should have no problem to follow them.
- Chapter 1 Introduction
- Chapter 2 Some Concepts in Time Series Analysis
- Chapter 3 Regime-Switching Models for Returns
- Chapter 4 Regime-Switching Models for Volatility
- Chapter 5 Artificial Neural Networks