1 Introduction . . . . . . . . . . . . . . . . . . . . . . . . . . . . . . . . . . . . . . . . . . 1
Part I Preliminaries
2 Data . . . . . . . . . . . . . . . . . . . . . . . . . . . . . . . . . . . . . . . . . . . . . . . . 7
3 Preprocessing . . . . . . . . . . . . . . . . . . . . . . . . . . . . . . . . . . . . . . . . . 13
3.1 Dealing with Noise . . . . . . . . . . . . . . . . . . . . . . . . . . . . . . . . . 13
3.2 Baseline Removal . . . . . . . . . . . . . . . . . . . . . . . . . . . . . . . . . . 18
3.3 Aligning Peaks—Warping . . . . . . . . . . . . . . . . . . . . . . . . . . . . 20
3.3.1 Parametric Time Warping . . . . . . . . . . . . . . . . . . . . . . 22
3.3.2 Dynamic Time Warping . . . . . . . . . . . . . . . . . . . . . . . 28
3.3.3 Practicalities . . . . . . . . . . . . . . . . . . . . . . . . . . . . . . . . 32
3.4 Peak Picking . . . . . . . . . . . . . . . . . . . . . . . . . . . . . . . . . . . . . 32
3.5 Scaling . . . . . . . . . . . . . . . . . . . . . . . . . . . . . . . . . . . . . . . . . . 34
3.6 Missing Data . . . . . . . . . . . . . . . . . . . . . . . . . . . . . . . . . . . . . 40
3.7 Conclusion . . . . . . . . . . . . . . . . . . . . . . . . . . . . . . . . . . . . . . . 40
Part II Exploratory Analysis
4 Principal Component Analysis . . . . . . . . . . . . . . . . . . . . . . . . . . . . 45
4.1 The Machinery . . . . . . . . . . . . . . . . . . . . . . . . . . . . . . . . . . . . 47
4.2 Doing It Yourself . . . . . . . . . . . . . . . . . . . . . . . . . . . . . . . . . . 48
4.3 Choosing the Number of PCs . . . . . . . . . . . . . . . . . . . . . . . . . 51
4.3.1 Scree Plots . . . . . . . . . . . . . . . . . . . . . . . . . . . . . . . . . 51
4.3.2 Statistical Tests . . . . . . . . . . . . . . . . . . . . . . . . . . . . . 51
4.4 Projections . . . . . . . . . . . . . . . . . . . . . . . . . . . . . . . . . . . . . . 53
4.5 R Functions for PCA . . . . . . . . . . . . . . . . . . . . . . . . . . . . . . . 55
4.6 Related Methods . . . . . . . . . . . . . . . . . . . . . . . . . . . . . . . . . . . 59
4.6.1 Multidimensional Scaling . . . . . . . . . . . . . . . . . . . . . . 59
4.6.2 Independent Component Analysis and Projection
Pursuit . . . . . . . . . . . . . . . . . . . . . . . . . . . . . . . . . . . . 62
4.6.3 Factor Analysis . . . . . . . . . . . . . . . . . . . . . . . . . . . . . 65
4.6.4 Discussion . . . . . . . . . . . . . . . . . . . . . . . . . . . . . . . . . 68
5 Self-Organizing Maps . . . . . . . . . . . . . . . . . . . . . . . . . . . . . . . . . . . 69
5.1 Training SOMs . . . . . . . . . . . . . . . . . . . . . . . . . . . . . . . . . . . . 70
5.2 Visualization . . . . . . . . . . . . . . . . . . . . . . . . . . . . . . . . . . . . . 72
5.3 Application . . . . . . . . . . . . . . . . . . . . . . . . . . . . . . . . . . . . . . . 76
5.4 R Packages for SOMs . . . . . . . . . . . . . . . . . . . . . . . . . . . . . . 77
5.5 Discussion . . . . . . . . . . . . . . . . . . . . . . . . . . . . . . . . . . . . . . . 79
6 Clustering . . . . . . . . . . . . . . . . . . . . . . . . . . . . . . . . . . . . . . . . . . . . 81
6.1 Hierarchical Clustering . . . . . . . . . . . . . . . . . . . . . . . . . . . . . . 82
6.2 Partitional Clustering. . . . . . . . . . . . . . . . . . . . . . . . . . . . . . . . 87
6.2.1 K-Means . . . . . . . . . . . . . . . . . . . . . . . . . . . . . . . . . . 87
6.2.2 K-Medoids . . . . . . . . . . . . . . . . . . . . . . . . . . . . . . . . . 89
6.3 Probabilistic Clustering . . . . . . . . . . . . . . . . . . . . . . . . . . . . . . 92
6.4 Comparing Clusterings . . . . . . . . . . . . . . . . . . . . . . . . . . . . . . 96
6.5 Discussion . . . . . . . . . . . . . . . . . . . . . . . . . . . . . . . . . . . . . . . 99
Part III Modelling
7 Classification . . . . . . . . . . . . . . . . . . . . . . . . . . . . . . . . . . . . . . . . . . 103
7.1 Discriminant Analysis . . . . . . . . . . . . . . . . . . . . . . . . . . . . . . . 105
7.1.1 Linear Discriminant Analysis . . . . . . . . . . . . . . . . . . . 106
7.1.2 Crossvalidation. . . . . . . . . . . . . . . . . . . . . . . . . . . . . . 110
7.1.3 Fisher LDA . . . . . . . . . . . . . . . . . . . . . . . . . . . . . . . . 111
7.1.4 Quadratic Discriminant Analysis . . . . . . . . . . . . . . . . . 115
7.1.5 Model-Based Discriminant Analysis . . . . . . . . . . . . . . 117
7.1.6 Regularized Forms of Discriminant Analysis . . . . . . . . 119
7.2 Nearest-Neighbor Approaches . . . . . . . . . . . . . . . . . . . . . . . . . 123
7.3 Tree-Based Approaches . . . . . . . . . . . . . . . . . . . . . . . . . . . . . . 127
7.3.1 Recursive Partitioning and Regression Trees . . . . . . . . 127
7.3.2 Discussion . . . . . . . . . . . . . . . . . . . . . . . . . . . . . . . . . 136
7.4 More Complicated Techniques. . . . . . . . . . . . . . . . . . . . . . . . . 136
7.4.1 Support Vector Machines . . . . . . . . . . . . . . . . . . . . . . 137
7.4.2 Artificial Neural Networks . . . . . . . . . . . . . . . . . . . . . 142
8 Multivariate Regression . . . . . . . . . . . . . . . . . . . . . . . . . . . . . . . . . 149
8.1 Multiple Regression . . . . . . . . . . . . . . . . . . . . . . . . . . . . . . . . 149
8.1.1 Limits of Multiple Regression . . . . . . . . . . . . . . . . . . . 151
8.2 PCR . . . . . . . . . . . . . . . . . . . . . . . . . . . . . . . . . . . . . . . . . . . . 153
8.2.1 The Algorithm . . . . . . . . . . . . . . . . . . . . . . . . . . . . . . 154
8.2.2 Selecting the Optimal Number of Components . . . . . . . 156
8.3 Partial Least Squares (PLS) Regression . . . . . . . . . . . . . . . . . . 160
8.3.1 The Algorithm(s) . . . . . . . . . . . . . . . . . . . . . . . . . . . . 161
8.3.2 Interpretation . . . . . . . . . . . . . . . . . . . . . . . . . . . . . . . 165
8.4 Ridge Regression . . . . . . . . . . . . . . . . . . . . . . . . . . . . . . . . . . 168
8.5 Continuum Methods . . . . . . . . . . . . . . . . . . . . . . . . . . . . . . . . 169
8.6 Some Non-linear Regression Techniques . . . . . . . . . . . . . . . . . 170
8.6.1 SVMs for Regression . . . . . . . . . . . . . . . . . . . . . . . . . 170
8.6.2 ANNs for Regression . . . . . . . . . . . . . . . . . . . . . . . . . 173
8.7 Classification as a Regression Problem. . . . . . . . . . . . . . . . . . . 175
8.7.1 Regression for LDA . . . . . . . . . . . . . . . . . . . . . . . . . . 176
8.7.2 PCDA . . . . . . . . . . . . . . . . . . . . . . . . . . . . . . . . . . . . 177
8.7.3 PLSDA . . . . . . . . . . . . . . . . . . . . . . . . . . . . . . . . . . . 182
8.7.4 Discussion . . . . . . . . . . . . . . . . . . . . . . . . . . . . . . . . . 184
Part IV Model Inspection
9 Validation . . . . . . . . . . . . . . . . . . . . . . . . . . . . . . . . . . . . . . . . . . . . 189
9.1 Representativity and Independence . . . . . . . . . . . . . . . . . . . . . . 190
9.2 Error Measures . . . . . . . . . . . . . . . . . . . . . . . . . . . . . . . . . . . . 192
9.3 Model Selection . . . . . . . . . . . . . . . . . . . . . . . . . . . . . . . . . . . 194
9.3.1 Permutation Approaches . . . . . . . . . . . . . . . . . . . . . . . 195
9.3.2 Model Selection Indices . . . . . . . . . . . . . . . . . . . . . . . 195
9.3.3 Including Model Selection in the Validation . . . . . . . . 196
9.4 Crossvalidation Revisited . . . . . . . . . . . . . . . . . . . . . . . . . . . . 197
9.4.1 LOO Crossvalidation . . . . . . . . . . . . . . . . . . . . . . . . . 197
9.4.2 Leave-Multiple-Out Crossvalidation. . . . . . . . . . . . . . . 199
9.4.3 Double Crossvalidation . . . . . . . . . . . . . . . . . . . . . . . . 199
9.5 The Jackknife . . . . . . . . . . . . . . . . . . . . . . . . . . . . . . . . . . . . . 200
9.6 The Bootstrap . . . . . . . . . . . . . . . . . . . . . . . . . . . . . . . . . . . . . 202
9.6.1 Error Estimation with the Bootstrap . . . . . . . . . . . . . . . 202
9.6.2 Confidence Intervals for Regression Coefficients . . . . . 206
9.6.3 Other R Packages for Bootstrapping . . . . . . . . . . . . . . 210
9.7 Integrated Modelling and Validation . . . . . . . . . . . . . . . . . . . . 211
9.7.1 Bagging . . . . . . . . . . . . . . . . . . . . . . . . . . . . . . . . . . . 211
9.7.2 Random Forests . . . . . . . . . . . . . . . . . . . . . . . . . . . . . 212
9.7.3 Boosting . . . . . . . . . . . . . . . . . . . . . . . . . . . . . . . . . . 217
10 Variable Selection . . . . . . . . . . . . . . . . . . . . . . . . . . . . . . . . . . . . . . 221
10.1 Coefficient Significance . . . . . . . . . . . . . . . . . . . . . . . . . . . . . . 222
10.1.1 Confidence Intervals for Individual Coefficients . . . . . . 223
10.1.2 Tests Based on Overall Error Contributions . . . . . . . . . 226
10.2 Explicit Coefficient Penalization. . . . . . . . . . . . . . . . . . . . . . . . 229
10.3 Global Optimization Methods . . . . . . . . . . . . . . . . . . . . . . . . . 232
10.3.1 Simulated Annealing . . . . . . . . . . . . . . . . . . . . . . . . . 234
10.3.2 Genetic Algorithms . . . . . . . . . . . . . . . . . . . . . . . . . . 241
10.3.3 Discussion . . . . . . . . . . . . . . . . . . . . . . . . . . . . . . . . . 245
Part V Applications
11 Chemometric Applications . . . . . . . . . . . . . . . . . . . . . . . . . . . . . . . 249
11.1 PCA in the Presence of Missing Values . . . . . . . . . . . . . . . . . . 250
11.1.1 Ignoring the Missing Values . . . . . . . . . . . . . . . . . . . . 252
11.1.2 Single Imputation . . . . . . . . . . . . . . . . . . . . . . . . . . . . 253
11.1.3 Multiple Imputation . . . . . . . . . . . . . . . . . . . . . . . . . . 255
11.2 Outlier Detection with Robust PCA . . . . . . . . . . . . . . . . . . . . . 256
11.2.1 Robust PCA. . . . . . . . . . . . . . . . . . . . . . . . . . . . . . . . 257
11.2.2 Discussion . . . . . . . . . . . . . . . . . . . . . . . . . . . . . . . . . 261
11.3 Multivariate Process Monitoring . . . . . . . . . . . . . . . . . . . . . . . 262
11.4 Orthogonal Signal Correction and OPLS . . . . . . . . . . . . . . . . . 266
11.5 Biomarker Identification . . . . . . . . . . . . . . . . . . . . . . . . . . . . . 269
11.6 Calibration Transfer . . . . . . . . . . . . . . . . . . . . . . . . . . . . . . . . 273
11.7 Batch Correction . . . . . . . . . . . . . . . . . . . . . . . . . . . . . . . . . . . 277
11.8 Multivariate Curve Resolution . . . . . . . . . . . . . . . . . . . . . . . . . 283
11.8.1 Theory . . . . . . . . . . . . . . . . . . . . . . . . . . . . . . . . . . . . 284
11.8.2 Finding Suitable Initial Estimates . . . . . . . . . . . . . . . . 284
11.8.3 Applying MCR . . . . . . . . . . . . . . . . . . . . . . . . . . . . . 288
11.8.4 Constraints . . . . . . . . . . . . . . . . . . . . . . . . . . . . . . . . . 290
11.8.5 Combining Data Sets . . . . . . . . . . . . . . . . . . . . . . . . . 293

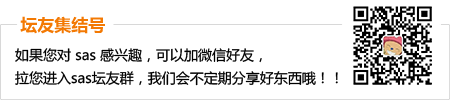