Random Forest
The algorithm to induce a random forest will create a bunch of random decision trees automatically. Since the trees are generated at random, most won't be all that meaningful to learning your classification/regression problem (maybe 99.9% of trees).
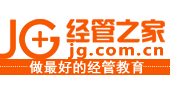
If an observation has a length of 45, blue eyes, and 2 legs, it's going to be classified as red.
Arboreal VotingSo what good are 10000 (probably) bad models? Well it turns out that they really aren't that helpful. But what is helpful are the few really good decision trees that you also generated along with the bad ones.
When you make a prediction, the new observation gets pushed down each decision tree and assigned a predicted value/label. Once each of the trees in the forest have reported its predicted value/label, the predictions are tallied up and the mode vote of all trees is returned as the final prediction.
Simply, the 99.9% of trees that are irrelevant make predictions that are all over the map and cancel each another out. The predictions of the minority of trees that are good top that noise and yield a good prediction.
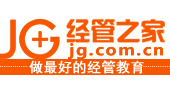
Random forest is the Leatherman of learning methods. You can throw pretty much anything at it and it'll do a serviceble job. It does a particularly good job of estimating inferred transformations, and, as a result, doesn't require much tuning like SVM (i.e. it's good for folks with tight deadlines).
An Example TransformationRandom forest is capable of learning without carefully crafted data transformations. Take the the f(x) = log(x) function for example.
Create some fake data and add a little noise.
import numpy as npx = np.random.uniform(1, 100, 1000)y = np.log(x) + np.random.normal(0, .3, 1000)full gist here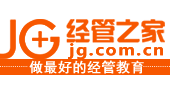
If we try and build a basic linear model to predict y using x we wind up with a straight line that sort of bisects the log(x) function. Whereas if we use a random forest, it does a much better job of approximating the log(x) curve and we get something that looks much more like the true function.
You could argue that the random forest overfits the log(x) function a little bit. Either way, I think this does a nice job of illustrating how the random forest isn't bound by linear constraints.
UsesVariable SelectionOne of the best use cases for random forest is feature selection. One of the byproducs of trying lots of decision tree variations is that you can examine which variables are working best/worst in each tree.
When a certain tree uses a one variable and another doesn't, you can compare the value lost or gained from the inclusion/exclusion of that variable. The good random forest implementations are going to do that for you, so all you need to do is know which method or variable to look at.
In the following examples, we're trying to figure out which variables are most important for classifying a wine as being red or white.
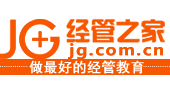
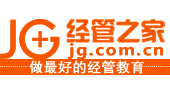
Random forest is also great for classification. It can be used to make predictions for categories with multiple possible values and it can be calibrated to output probabilities as well. One thing you do need to watch out for is overfitting. Random forest can be prone to overfitting, especially when working with relatively small datasets. You should be suspicious if your model is making "too good" of predictions on our test set.
One way to overfitting is to only use really relevant features in your model. While this isn't always cut and dry, using a feature selection technique (like the one mentioned previously) can make it a lot easier.
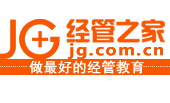
Yep. It does regression too.
I've found that random forest--unlike other algorithms--does really well learning on categorical variables or a mixture of categorical and real variables. Categorical variables with high cardinality (# of possible values) can be tricky, so having something like this in your back pocket can come in quite useful.
A Short Python ExampleScikit-Learn is a great way to get started with random forest. The scikit-learn API is extremely consistent across algorithms, so you horse race and switch between models very easily. A lot of times I start with something simple and then move to random forest.
One of the best features of the random forest implementation in scikit-learn is the n_jobsparameter. This will automatically paralellize fitting your random forest based on the number of cores you want to use. Here's a great presentation by scikit-learn contributor Olivier Grisel where he talks about training a random forest on a 20 node EC2 cluster.
from sklearn.datasets import load_iris from sklearn.ensemble import RandomForestClassifier import pandas as pd import numpy as np iris = load_iris() df = pd.DataFrame(iris.data, columns=iris.feature_names) df['is_train'] = np.random.uniform(0, 1, len(df)) <= .75 df['species'] = pd.Factor(iris.target, iris.target_names) df.head() train, test = df[df['is_train']==True], df[df['is_train']==False] features = df.columns[:4] clf = RandomForestClassifier(n_jobs=2) y, _ = pd.factorize(train['species']) clf.fit(train[features], y) preds = iris.target_names[clf.predict(test[features])] pd.crosstab(test['species'], preds, rownames=['actual'], colnames=['preds']) |
view rawrf_iris.py hosted with ❤ by GitHub
Looks pretty good!
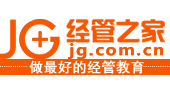
Random forests are remarkabley easy to use given how advanced they are. As with any modeling, be wary of overfitting. If you're interested in getting started with random forest inR, check out the randomForest package.