There has been a lot of buzz surrounding the potential impact deep learning will have in the field of analytics. This post looks at the origins of deep learning.
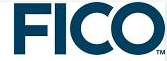
Due to the recent acquisition of DeepMind by Google for an estimated $500+ million, and the movement of some academic experts to high-profile tech giants, there has been a lot of buzz surrounding the potential impact deep learning will have in the field of analytics. At FICO, we’re excited about this emerging machine learning technology and want to share how we think it fits into the world of analytics.
Many advances in analytics and machine learning have been based on our understanding of how the brain works. Deep learning is no exception — it takes its inspiration from our understanding of the cortex in the brain. The brain has many regions which form a hierarchy of processing, where sensory data flows from one region to another, being transformed and combined with other information along the way. While it may seem instantaneous when we recognize a face or a voice, there are actually many stages of processing between our senses and a set of neurons that we can clearly link to that particular person.
This is the same process used in neural network models — such as those FICO uses in fraud detection. These networks have an input layer (raw data or derived features), “hidden” layers that process and combines the inputs, and an output layer (such as a score that indicates fraud risk).
Recognizing a complex object involves first recognizing and processing a large number of features. For example, useful features for an image recognition network include edges, corners and colored regions. A combination of features will lead to recognition of a larger pattern. For example, certain combinations of edges, corners and colors would indicate a human face.
In a financial decision problem like fraud detection, a feature might be the amount of a transaction, or the kind of retailer involved — something that can be clearly identified. A certain number of transaction amounts, at a certain frequency with certain types of retailers, will indicate a higher fraud risk.
Shallow Learning (Fraud Example)
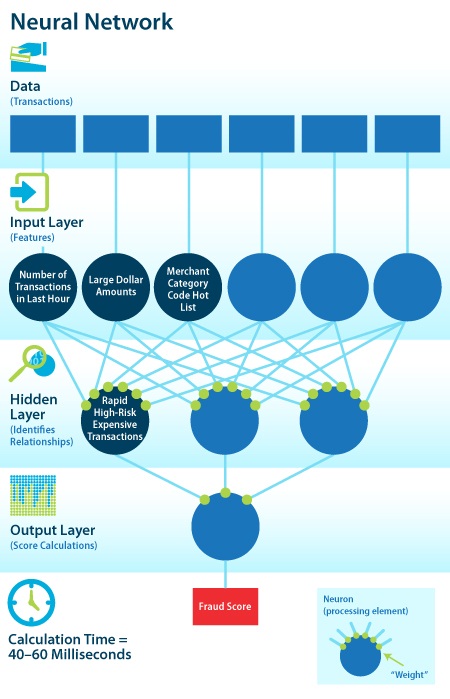
For some problems in analytics, we rely on huge data stores (such as the FICO fraud data consortium) to craft the most appropriate features and combinations of features for our models. For instance, over 20 years in studying fraud data, we know what kinds of relationships we’re looking for, so we can build a single “hidden” layer that processes raw transaction data to detect fraud extremely efficiently, allowing for calculations of fraud risk in 40-60 milliseconds (about 1/5h the time it takes you to blink). Such hand-crafted features can lead to robust models that can make quick decisions. This is why, for many years, most artificial neural network research was focused on networks with a single layer of processing. These are sometimes called shallow networks.
However, deep learning research has shown many new ways to let the mass of Big Data determine the most important features for a decision task. Deep learning happens in deep neural networks, where the deep refers to the factor that multiple layers of processing transform the input data (whether it’s images, speech or text) into some output useful for making decisions (perhaps whether a certain object is in a security camera image, or inferring context from text).
An example of this kind of deep learning is discussed in The Analytics Store’s blog entry on deep learning. Per that post, “The image below shows a simplified illustration of this where a stack of neural networks are used to classify images. While the data presented to the network would be raw pixel values, internally the network would generate much higher level features.”
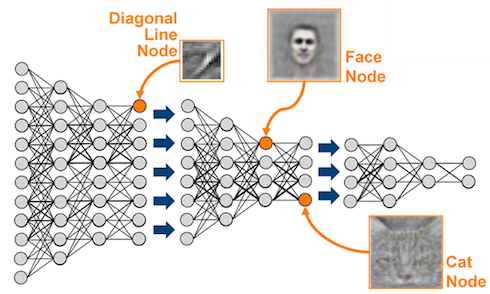
Source: http://theanalyticsstore.com/deep-learning
Over time algorithms and research have advanced to allow more layers to be added to neural models to more closely mimic the complexity of the brain. With the explosion of Big Data, there are unique areas to apply these techniques where simpler features build into more complex features.
Certainly there’s a lot of variety to deep learning algorithms, and we’re likely to see many new variations over the next years as more applications are developed. The current crop of deep learning is drawing on only a fraction of what is known about real neurons and brains, indicating huge potential for this line of scientific exploration.
Scott Zoldi is a VP, Analytic Science at FICO.