[Springer Use R系列最新书籍] Analyzing Compositional Data with R
发布:kaifengedu | 分类:考研
关于本站
人大经济论坛-经管之家:分享大学、考研、论文、会计、留学、数据、经济学、金融学、管理学、统计学、博弈论、统计年鉴、行业分析包括等相关资源。
经管之家是国内活跃的在线教育咨询平台!
经管之家新媒体交易平台
提供"微信号、微博、抖音、快手、头条、小红书、百家号、企鹅号、UC号、一点资讯"等虚拟账号交易,真正实现买卖双方的共赢。【请点击这里访问】
期刊
- 期刊库 | 马上cssci就要更新 ...
- 期刊库 | 【独家发布】《财 ...
- 期刊库 | 【独家发布】“我 ...
- 期刊库 | 【独家发布】“我 ...
- 期刊库 | 【独家发布】国家 ...
- 期刊库 | 请问Management S ...
- 期刊库 | 英文期刊库
- 核心期刊 | 歧路彷徨:核心期 ...
TOP热门关键词
书名:AnalyzingCompositionalDatawithR目录及书回复可见.Contents1Introduction..................................................................11.1WhatAreCompositionalData?............................... ...
坛友互助群![]() |
扫码加入各岗位、行业、专业交流群![]() |
目录及书回复可见.
Contents
1 Introduction . . . . . . . . . . . . . . . . . . . . . . . . . . . . . . . . . . . . . . . . . . . . . . . . . . . . . . . . . . . . . . . . . . 1
1.1 What Are Compositional Data? . . . . . . . . . . . . . . . . . . . . . . . . . . . . . . . . . . . . . . . . 1
1.1.1 Compositions Are Portions of a Total . . . . . . . . . . . . . . . . . . . . . . . . 1
1.1.2 Compositions Are Multivariate by Nature . . . . . . . . . . . . . . . . . . . 3
1.1.3 The Total Sum of a Composition Is Irrelevant . . . . . . . . . . . . . . . 3
1.1.4 Practically All Compositions Are Subcompositions.. . . . . . . . 4
1.1.5 The Very Brief History of Compositional Data Analysis. . . . 5
1.1.6 Software for Compositional Data Analysis . . . . . . . . . . . . . . . . . . 6
1.2 Getting Started with R . . . . . . . . . . . . . . . . . . . . . . . . . . . . . . . . . . . . . . . . . . . . . . . . . . 6
1.2.1 Software Needed for the Examples in This Book. . . . . . . . . . . . 6
1.2.2 Installing R and the Extension Packages .. . . . . . . . . . . . . . . . . . . . 7
1.2.3 Basic R Usage . . . . . . . . . . . . . . . . . . . . . . . . . . . . . . . . . . . . . . . . . . . . . . . . . 7
1.2.4 Getting Help for Composition Specific Commands . . . . . . . . . 10
1.2.5 Troubleshooting . . . . . . . . . . . . . . . . . . . . . . . . . . . . . . . . . . . . . . . . . . . . . . . 11
References . . . . . . . . . . . . . . . . . . . . . . . . . . . . . . . . . . . . . . . . . . . . . . . . . . . . . . . . . . . . . . . . . . . . . 11
2 Fundamental Concepts of Compositional Data Analysis . . . . . . . . . . . . . . . . 13
2.1 A Practical View to Compositional Concepts . . . . . . . . . . . . . . . . . . . . . . . . . 13
2.1.1 Definition of Compositional Data . . . . . . . . . . . . . . . . . . . . . . . . . . . . 13
2.1.2 Subcompositions and the Closure Operation.. . . . . . . . . . . . . . . . 14
2.1.3 Completion of a Composition . . . . . . . . . . . . . . . . . . . . . . . . . . . . . . . . 15
2.1.4 Compositions as Equivalence Classes . . . . . . . . . . . . . . . . . . . . . . . . 17
2.1.5 Perturbation as a Change of Units . . . . . . . . . . . . . . . . . . . . . . . . . . . . 18
2.1.6 Amalgamation . . . . . . . . . . . . . . . . . . . . . . . . . . . . . . . . . . . . . . . . . . . . . . . . . 19
2.1.7 Missing Values and Outliers . . . . . . . . . . . . . . . . . . . . . . . . . . . . . . . . . . 20
2.2 Principles of Compositional Analysis . . . . . . . . . . . . . . . . . . . . . . . . . . . . . . . . . . 20
2.2.1 Scaling Invariance .. . . . . . . . . . . . . . . . . . . . . . . . . . . . . . . . . . . . . . . . . . . . 20
2.2.2 Perturbation Invariance.. . . . . . . . . . . . . . . . . . . . . . . . . . . . . . . . . . . . . . . 21
2.2.3 Subcompositional Coherence .. . . . . . . . . . . . . . . . . . . . . . . . . . . . . . . . 22
2.2.4 Permutation Invariance .. . . . . . . . . . . . . . . . . . . . . . . . . . . . . . . . . . . . . . . 23
2.3 Elementary Compositional Graphics . . . . . . . . . . . . . . . . . . . . . . . . . . . . . . . . . . . 23
2.3.1 Sense and Nonsense of Scatterplots of Components . . . . . . . . 24
2.3.2 Ternary Diagrams . . . . . . . . . . . . . . . . . . . . . . . . . . . . . . . . . . . . . . . . . . . . . 24
2.3.3 Log-Ratio Scatterplots . . . . . . . . . . . . . . . . . . . . . . . . . . . . . . . . . . . . . . . . 27
2.3.4 Bar Plots and Pie Charts . . . . . . . . . . . . . . . . . . . . . . . . . . . . . . . . . . . . . . 28
2.4 Multivariate Scales . . . . . . . . . . . . . . . . . . . . . . . . . . . . . . . . . . . . . . . . . . . . . . . . . . . . . . 29
2.4.1 Classical Multivariate Vectorial Data (rmult) . . . . . . . . . . . . . . . . 31
2.4.2 Positive Data with Absolute Geometry (rplus).. . . . . . . . . . . . . . 31
2.4.3 Positive Data with Relative Geometry (aplus) . . . . . . . . . . . . . . . 32
2.4.4 Compositional Data with Absolute Geometry (rcomp).. . . . . 32
2.4.5 Compositional Data with Aitchison Geometry (acomp) . . . . 33
2.4.6 Count Compositions (ccomp).. . . . . . . . . . . . . . . . . . . . . . . . . . . . . . . . 34
2.4.7 Practical Considerations on Scale Selection . . . . . . . . . . . . . . . . . 34
2.5 The Aitchison Simplex . . . . . . . . . . . . . . . . . . . . . . . . . . . . . . . . . . . . . . . . . . . . . . . . . 37
2.5.1 The Simplex and the Closure Operation . . . . . . . . . . . . . . . . . . . . . 37
2.5.2 Perturbation as Compositional Sum . . . . . . . . . . . . . . . . . . . . . . . . . . 37
2.5.3 Powering as Compositional Scalar Multiplication .. . . . . . . . . . 39
2.5.4 Compositional Scalar Product, Norm, and Distance. . . . . . . . . 39
2.5.5 The Centered Log-Ratio Transformation (clr) . . . . . . . . . . . . . . . 41
2.5.6 The Isometric Log-Ratio Transformation (ilr) . . . . . . . . . . . . . . . 42
2.5.7 The Additive Log-Ratio Transformation (alr) . . . . . . . . . . . . . . . 44
2.5.8 Geometric Representation of Statistical Results . . . . . . . . . . . . . 45
2.5.9 Expectation and Variance in the Simplex . . . . . . . . . . . . . . . . . . . . 47
References . . . . . . . . . . . . . . . . . . . . . . . . . . . . . . . . . . . . . . . . . . . . . . . . . . . . . . . . . . . . . . . . . . . . . 49
3 Distributions for Random Compositions . . . . . . . . . . . . . . . . . . . . . . . . . . . . . . . . . . 51
3.1 Continuous Distribution Models . . . . . . . . . . . . . . . . . . . . . . . . . . . . . . . . . . . . . . . 51
3.1.1 The Normal Distribution on the Simplex.. . . . . . . . . . . . . . . . . . . . 51
3.1.2 Testing for Compositional Normality . . . . . . . . . . . . . . . . . . . . . . . . 53
3.1.3 The Dirichlet Distribution. . . . . . . . . . . . . . . . . . . . . . . . . . . . . . . . . . . . . 58
3.1.4 The Aitchison Distribution. . . . . . . . . . . . . . . . . . . . . . . . . . . . . . . . . . . . 61
3.2 Models for Count Compositions . . . . . . . . . . . . . . . . . . . . . . . . . . . . . . . . . . . . . . . 62
3.2.1 The Multinomial Distribution.. . . . . . . . . . . . . . . . . . . . . . . . . . . . . . . . 62
3.2.2 The Multi-Poisson Distribution . . . . . . . . . . . . . . . . . . . . . . . . . . . . . . . 64
3.2.3 Double Stochastic Count Distributions. . . . . . . . . . . . . . . . . . . . . . . 66
3.3 Relations Between Distributions . . . . . . . . . . . . . . . . . . . . . . . . . . . . . . . . . . . . . . . 67
3.3.1 Marginalization Properties . . . . . . . . . . . . . . . . . . . . . . . . . . . . . . . . . . . . 67
3.3.2 Conjugated Priors . . . . . . . . . . . . . . . . . . . . . . . . . . . . . . . . . . . . . . . . . . . . . 70
4 Descriptive Analysis of Compositional Data . . . . . . . . . . . . . . . . . . . . . . . . . . . . . . 73
4.1 Descriptive Statistics . . . . . . . . . . . . . . . . . . . . . . . . . . . . . . . . . . . . . . . . . . . . . . . . . . . . 73
4.1.1 CompositionalMean . . . . . . . . . . . . . . . . . . . . . . . . . . . . . . . . . . . . . . . . . . 74
4.1.2 Metric Variance and Standard Deviation .. . . . . . . . . . . . . . . . . . . . 75
4.1.3 Variation Matrix and Its Relatives . . . . . . . . . . . . . . . . . . . . . . . . . . . . 76
4.1.4 Variance Matrices . . . . . . . . . . . . . . . . . . . . . . . . . . . . . . . . . . . . . . . . . . . . . 80
4.1.5 Normal-Based Predictive and Confidence Regions . . . . . . . . . . 82
4.2 ExploringMarginals . . . . . . . . . . . . . . . . . . . . . . . . . . . . . . . . . . . . . . . . . . . . . . . . . . . . 85
4.2.1 The Three Types of Compositional Marginals .. . . . . . . . . . . . . . 85
4.2.2 Ternary Diagram Matrices for Multidimensional
Compositions . . . . . . . . . . . . . . . . . . . . . . . . . . . . . . . . . . . . . . . . . . . . . . . . . . 87
4.3 Exploring Projections .. . . . . . . . . . . . . . . . . . . . . . . . . . . . . . . . . . . . . . . . . . . . . . . . . . 89
4.3.1 Projections and Balances. . . . . . . . . . . . . . . . . . . . . . . . . . . . . . . . . . . . . . 89
4.3.2 Balance Bases . . . . . . . . . . . . . . . . . . . . . . . . . . . . . . . . . . . . . . . . . . . . . . . . . 91
4.3.3 The Coda-Dendrogram .. . . . . . . . . . . . . . . . . . . . . . . . . . . . . . . . . . . . . . . 92
References . . . . . . . . . . . . . . . . . . . . . . . . . . . . . . . . . . . . . . . . . . . . . . . . . . . . . . . . . . . . . . . . . . . . . 93
5 Linear Models for Compositions . . . . . . . . . . . . . . . . . . . . . . . . . . . . . . . . . . . . . . . . . . . 95
5.1 Introduction . . . . . . . . . . . . . . . . . . . . . . . . . . . . . . . . . . . . . . . . . . . . . . . . . . . . . . . . . . . . . 95
5.1.1 Classical Linear Regression (Continuous Covariables) . . . . . 95
5.1.2 Classical Analysis of the Variance
(Discrete Covariables).. . . . . . . . . . . . . . . . . . . . . . . . . . . . . . . . . . . . . . . . 100
5.1.3 The Different Types of Compositional
Regression and ANOVA . . . . . . . . . . . . . . . . . . . . . . . . . . . . . . . . . . . . . . 102
5.2 Compositions as Independent Variables . . . . . . . . . . . . . . . . . . . . . . . . . . . . . . . 103
5.2.1 Example.. . . . . . . . . . . . . . . . . . . . . . . . . . . . . . . . . . . . . . . . . . . . . . . . . . . . . . . 103
5.2.2 Direct Visualization of the Dependence .. . . . . . . . . . . . . . . . . . . . . 103
5.2.3 The Model . . . . . . . . . . . . . . . . . . . . . . . . . . . . . . . . . . . . . . . . . . . . . . . . . . . . . 106
5.2.4 Estimation of Regression Parameters. . . . . . . . . . . . . . . . . . . . . . . . . 107
5.2.5 Displaying the Model . . . . . . . . . . . . . . . . . . . . . . . . . . . . . . . . . . . . . . . . . 108
5.2.6 Prediction and Predictive Regions . . . . . . . . . . . . . . . . . . . . . . . . . . . . 110
5.2.7 Model Checks . . . . . . . . . . . . . . . . . . . . . . . . . . . . . . . . . . . . . . . . . . . . . . . . . 112
5.2.8 The Strength of the Relationship . . . . . . . . . . . . . . . . . . . . . . . . . . . . . 112
5.2.9 Global and Individual Tests . . . . . . . . . . . . . . . . . . . . . . . . . . . . . . . . . . . 114
5.2.10 Model Diagnostic Plots . . . . . . . . . . . . . . . . . . . . . . . . . . . . . . . . . . . . . . . 115
5.2.11 Checking the Normality Assumptions . . . . . . . . . . . . . . . . . . . . . . . 117
5.2.12 Checking Constant Variance .. . . . . . . . . . . . . . . . . . . . . . . . . . . . . . . . . 118
5.2.13 Robust Regression. . . . . . . . . . . . . . . . . . . . . . . . . . . . . . . . . . . . . . . . . . . . . 118
5.2.14 Quadratic Regression. . . . . . . . . . . . . . . . . . . . . . . . . . . . . . . . . . . . . . . . . . 120
5.3 Compositions as Dependent Variables . . . . . . . . . . . . . . . . . . . . . . . . . . . . . . . . . 122
5.3.1 Example.. . . . . . . . . . . . . . . . . . . . . . . . . . . . . . . . . . . . . . . . . . . . . . . . . . . . . . . 122
5.3.2 Direct Visualization of the Dependence .. . . . . . . . . . . . . . . . . . . . . 125
5.3.3 The Model and Its R Interface . . . . . . . . . . . . . . . . . . . . . . . . . . . . . . . . 129
5.3.4 Estimation and Representation of the Model Parameters . . . 134
5.3.5 Testing the Influence of Each Variable . . . . . . . . . . . . . . . . . . . . . . . 138
5.3.6 Comparing Predicted and Observed Values . . . . . . . . . . . . . . . . . . 140
5.3.7 Prediction of New Values . . . . . . . . . . . . . . . . . . . . . . . . . . . . . . . . . . . . . 142
5.3.8 Residuals . . . . . . . . . . . . . . . . . . . . . . . . . . . . . . . . . . . . . . . . . . . . . . . . . . . . . . . 145
5.3.9 Measures of Compositional Determination . . . . . . . . . . . . . . . . . . 152
5.4 Compositions as Both Dependent and Independent Variables .. . . . . . . 154
5.4.1 Example.. . . . . . . . . . . . . . . . . . . . . . . . . . . . . . . . . . . . . . . . . . . . . . . . . . . . . . . 154
5.4.2 Visualization .. . . . . . . . . . . . . . . . . . . . . . . . . . . . . . . . . . . . . . . . . . . . . . . . . . 155
5.4.3 The Composition-to-Composition Regression Model .. . . . . . 155
5.4.4 Representation and Visualization of Coefficients .. . . . . . . . . . . 157
5.5 Advanced Considerations.. . . . . . . . . . . . . . . . . . . . . . . . . . . . . . . . . . . . . . . . . . . . . . 161
7.3 Outliers . . . . . . . . . . . . . . . . . . . . . . . . . . . . . . . . . . . . . . . . . . . . . . . . . . . . . . . . . . . . . . . . . . 237
7.3.1 Background on Outliers . . . . . . . . . . . . . . . . . . . . . . . . . . . . . . . . . . . . . . . 237
7.3.2 Types of Outliers . . . . . . . . . . . . . . . . . . . . . . . . . . . . . . . . . . . . . . . . . . . . . . 240
7.3.3 Robust Estimation . . . . . . . . . . . . . . . . . . . . . . . . . . . . . . . . . . . . . . . . . . . . . 241
7.3.4 Detection and Classification of Outliers. . . . . . . . . . . . . . . . . . . . . . 241
7.4 Descriptive Analysis of Outliers . . . . . . . . . . . . . . . . . . . . . . . . . . . . . . . . . . . . . . . 242
7.4.1 Robust Estimation of Mean and Variance .. . . . . . . . . . . . . . . . . . . 242
7.4.2 Detecting Outliers . . . . . . . . . . . . . . . . . . . . . . . . . . . . . . . . . . . . . . . . . . . . . 243
7.4.3 Counting Outliers . . . . . . . . . . . . . . . . . . . . . . . . . . . . . . . . . . . . . . . . . . . . . 246
7.4.4 Classifying Outliers . . . . . . . . . . . . . . . . . . . . . . . . . . . . . . . . . . . . . . . . . . . 247
7.5 Working with Outliers . . . . . . . . . . . . . . . . . . . . . . . . . . . . . . . . . . . . . . . . . . . . . . . . . . 249
7.5.1 Random Outliers . . . . . . . . . . . . . . . . . . . . . . . . . . . . . . . . . . . . . . . . . . . . . . 249
7.5.2 Failed Measurement or Transmission
in Individual Components.. . . . . . . . . . . . . . . . . . . . . . . . . . . . . . . . . . . . 249
7.5.3 Subpopulations . . . . . . . . . . . . . . . . . . . . . . . . . . . . . . . . . . . . . . . . . . . . . . . . 250
7.5.4 Polluted Samples . . . . . . . . . . . . . . . . . . . . . . . . . . . . . . . . . . . . . . . . . . . . . . 251
7.5.5 Heavy-Tailed Compositional Distributions . . . . . . . . . . . . . . . . . . 252
References . . . . . . . . . . . . . . . . . . . . . . . . . . . . . . . . . . . . . . . . . . . . . . . . . . . . . . . . . . . . . . . . . . . . . 253
Index . . . . . . . . . . . . . . . . . . . . . . . . . . . . . . . . . . . . . . . . . . . . . . . . . . . . . . . . . . . . . . . . . . . . . . . . . . . . . . . 255
[hide][/hide]
扫码或添加微信号:坛友素质互助
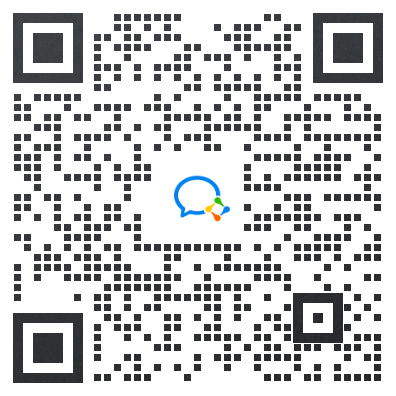
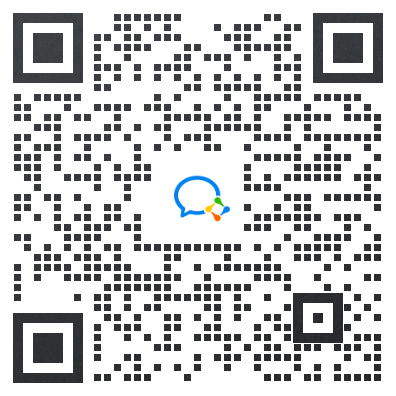
「经管之家」APP:经管人学习、答疑、交友,就上经管之家!
免流量费下载资料----在经管之家app可以下载论坛上的所有资源,并且不额外收取下载高峰期的论坛币。
涵盖所有经管领域的优秀内容----覆盖经济、管理、金融投资、计量统计、数据分析、国贸、财会等专业的学习宝库,各类资料应有尽有。
来自五湖四海的经管达人----已经有上千万的经管人来到这里,你可以找到任何学科方向、有共同话题的朋友。
经管之家(原人大经济论坛),跨越高校的围墙,带你走进经管知识的新世界。
扫描下方二维码下载并注册APP
免流量费下载资料----在经管之家app可以下载论坛上的所有资源,并且不额外收取下载高峰期的论坛币。
涵盖所有经管领域的优秀内容----覆盖经济、管理、金融投资、计量统计、数据分析、国贸、财会等专业的学习宝库,各类资料应有尽有。
来自五湖四海的经管达人----已经有上千万的经管人来到这里,你可以找到任何学科方向、有共同话题的朋友。
经管之家(原人大经济论坛),跨越高校的围墙,带你走进经管知识的新世界。
扫描下方二维码下载并注册APP

您可能感兴趣的文章
本站推荐的文章
人气文章
本文标题:[Springer Use R系列最新书籍] Analyzing Compositional Data with R
本文链接网址:https://bbs.pinggu.org/jg/kaoyankaobo_kaoyan_2599341_1.html
2.转载的文章仅代表原创作者观点,与本站无关。其原创性以及文中陈述文字和内容未经本站证实,本站对该文以及其中全部或者部分内容、文字的真实性、完整性、及时性,不作出任何保证或承若;
3.如本站转载稿涉及版权等问题,请作者及时联系本站,我们会及时处理。