挖掘FDA药品标签
发布:qililaimend | 分类:考研
关于本站
人大经济论坛-经管之家:分享大学、考研、论文、会计、留学、数据、经济学、金融学、管理学、统计学、博弈论、统计年鉴、行业分析包括等相关资源。
经管之家是国内活跃的在线教育咨询平台!
经管之家新媒体交易平台
提供"微信号、微博、抖音、快手、头条、小红书、百家号、企鹅号、UC号、一点资讯"等虚拟账号交易,真正实现买卖双方的共赢。【请点击这里访问】
期刊
- 期刊库 | 马上cssci就要更新 ...
- 期刊库 | 【独家发布】《财 ...
- 期刊库 | 【独家发布】“我 ...
- 期刊库 | 【独家发布】“我 ...
- 期刊库 | 【独家发布】国家 ...
- 期刊库 | 请问Management S ...
- 期刊库 | 英文期刊库
- 核心期刊 | 歧路彷徨:核心期 ...
TOP热门关键词
还是我自己的文章挖掘FDA药品标签文本挖掘,医疗管理AbstractBackground:CincinnatiChildren’sHospitalMedicalCenter(CCHMC)hasbuilttheinitialNaturalLanguageProcessing(NLP)componenttoextractmedicationswithth ...
坛友互助群![]() |
扫码加入各岗位、行业、专业交流群![]() |
挖掘FDA药品标签
文本挖掘,
医疗管理
Abstract
Background: Cincinnati Children’s Hospital Medical Center (CCHMC) has built the initial Natural Language
Processing (NLP) component to extract medications with their corresponding medical conditions (Indications,
Contraindications, Overdosage, and Adverse Reactions) as triples of medication-related information ([(1) drug
name]-[(2) medical condition]-[(3) LOINC section header]) for an intelligent database system, in order to improve
patient safety and the quality of health care. The Food and Drug Administration’s (FDA) drug labels are used to
demonstrate the feasibility of building the triples as an intelligent database system task.
Methods: This paper discusses a hybrid NLP system, called AutoMCExtractor, to collect medical conditions
(including disease/disorder and sign/symptom) from drug labels published by the FDA. Altogether, 6,611 medical
conditions in a manually-annotated gold standard were used for the system evaluation. The pre-processing step
extracted the plain text from XML file and detected eight related LOINC sections (e.g. Adverse Reactions, Warnings
and Precautions) for medical condition extraction. Conditional Random Fields (CRF) classifiers, trained on token,
linguistic, and semantic features, were then used for medical condition extraction. Lastly, dictionary-based postprocessing
corrected boundary-detection errors of the CRF step. We evaluated the AutoMCExtractor on manuallyannotated
FDA drug labels and report the results on both token and span levels.
Results: Precision, recall, and F-measure were 0.90, 0.81, and 0.85, respectively, for the span level exact match; for
the token-level evaluation, precision, recall, and F-measure were 0.92, 0.73, and 0.82, respectively.
Conclusions: The results demonstrate that (1) medical conditions can be extracted from FDA drug labels with high
performance; and (2) it is feasible to develop a framework for an intelligent database system.
Keywords: Medical condition, Disease and disorders, Sign and symptoms, cTAKES, NLP, Natural language
processing, IE, Information extraction, CRF, Conditional random fields, FDA drug labels
扫码或添加微信号:坛友素质互助
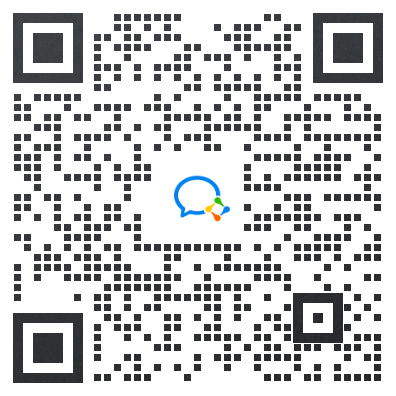
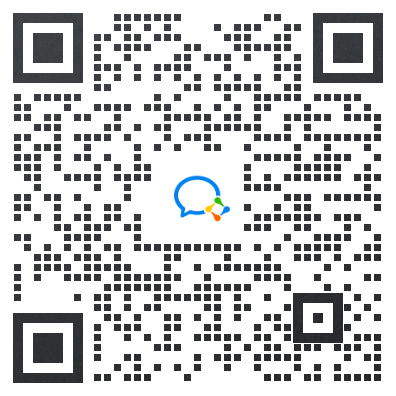
「经管之家」APP:经管人学习、答疑、交友,就上经管之家!
免流量费下载资料----在经管之家app可以下载论坛上的所有资源,并且不额外收取下载高峰期的论坛币。
涵盖所有经管领域的优秀内容----覆盖经济、管理、金融投资、计量统计、数据分析、国贸、财会等专业的学习宝库,各类资料应有尽有。
来自五湖四海的经管达人----已经有上千万的经管人来到这里,你可以找到任何学科方向、有共同话题的朋友。
经管之家(原人大经济论坛),跨越高校的围墙,带你走进经管知识的新世界。
扫描下方二维码下载并注册APP
免流量费下载资料----在经管之家app可以下载论坛上的所有资源,并且不额外收取下载高峰期的论坛币。
涵盖所有经管领域的优秀内容----覆盖经济、管理、金融投资、计量统计、数据分析、国贸、财会等专业的学习宝库,各类资料应有尽有。
来自五湖四海的经管达人----已经有上千万的经管人来到这里,你可以找到任何学科方向、有共同话题的朋友。
经管之家(原人大经济论坛),跨越高校的围墙,带你走进经管知识的新世界。
扫描下方二维码下载并注册APP

您可能感兴趣的文章
本站推荐的文章
人气文章
1.凡人大经济论坛-经管之家转载的文章,均出自其它媒体或其他官网介绍,目的在于传递更多的信息,并不代表本站赞同其观点和其真实性负责;
2.转载的文章仅代表原创作者观点,与本站无关。其原创性以及文中陈述文字和内容未经本站证实,本站对该文以及其中全部或者部分内容、文字的真实性、完整性、及时性,不作出任何保证或承若;
3.如本站转载稿涉及版权等问题,请作者及时联系本站,我们会及时处理。
2.转载的文章仅代表原创作者观点,与本站无关。其原创性以及文中陈述文字和内容未经本站证实,本站对该文以及其中全部或者部分内容、文字的真实性、完整性、及时性,不作出任何保证或承若;
3.如本站转载稿涉及版权等问题,请作者及时联系本站,我们会及时处理。