关于本站
人大经济论坛-经管之家:分享大学、考研、论文、会计、留学、数据、经济学、金融学、管理学、统计学、博弈论、统计年鉴、行业分析包括等相关资源。
经管之家是国内活跃的在线教育咨询平台!
经管之家新媒体交易平台
提供"微信号、微博、抖音、快手、头条、小红书、百家号、企鹅号、UC号、一点资讯"等虚拟账号交易,真正实现买卖双方的共赢。【请点击这里访问】
期刊
- 期刊库 | 马上cssci就要更新 ...
- 期刊库 | 【独家发布】《财 ...
- 期刊库 | 【独家发布】“我 ...
- 期刊库 | 【独家发布】“我 ...
- 期刊库 | 【独家发布】国家 ...
- 期刊库 | 请问Management S ...
- 期刊库 | 英文期刊库
- 核心期刊 | 歧路彷徨:核心期 ...
TOP热门关键词
PredictiveAnalytics:ThePowertoPredictWhoWillClick,Buy,Lie,orDie,RevisedandUpdatedEricSiegel"Mesmerizing&fascinating..."—TheSeattlePost-Intelligencer"TheFreakonomicsofbigdata."—SteinKretsin ...
坛友互助群![]() |
扫码加入各岗位、行业、专业交流群![]() |
Eric Siegel
"Mesmerizing & fascinating..." —The Seattle Post-Intelligencer
"The Freakonomics of big data." —Stein Kretsinger, founding executive of Advertising.com
Award-winning | Used by over 30 universities | Translated into 9 languages
An introduction for everyone. In this rich, fascinating — surprisingly accessible — introduction, leading expert Eric Siegel reveals how predictive analytics works, and how it affects everyone every day. Rather than a “how to” for hands-on techies, the book serves lay readers and experts alike by covering new case studies and the latest state-of-the-art techniques.
Prediction is booming. It reinvents industries and runs the world. Companies, governments, law enforcement, hospitals, and universities are seizing upon the power. These institutions predict whether you're going to click, buy, lie, or die.
Why? For good reason: predicting human behavior combats risk, boosts sales, fortifies healthcare, streamlines manufacturing, conquers spam, optimizes social networks, toughens crime fighting, and wins elections.
How? Prediction is powered by the world's most potent, flourishing unnatural resource: data. Accumulated in large part as the by-product of routine tasks, data is the unsalted, flavorless residue deposited en masse as organizations churn away. Surprise! This heap of refuse is a gold mine. Big data embodies an extraordinary wealth of experience from which to learn.
Predictive Analytics unleashes the power of data. With this technology, the computer literally learns from data how to predict the future behavior of individuals. Perfect prediction is not possible, but putting odds on the future drives millions of decisions more effectively, determining whom to call, mail, investigate, incarcerate, set up on a date, or medicate.
In this lucid, captivating introduction — now in its Revised and Updated edition — former Columbia University professor and Predictive Analytics World founder Eric Siegel reveals the power and perils of prediction:
• What type of mortgage risk Chase Bank predicted before the recession.
• Predicting which people will drop out of school, cancel a subscription, or get divorced before they even know it themselves.
• Why early retirement predicts a shorter life expectancy and vegetarians miss fewer flights.
• Five reasons why organizations predict death — including one health insurance company.
• How U.S. Bank and Obama for America calculated — and Hillary for America 2016 plans to calculate — the way to most strongly persuade each individual.
• Why the NSA wants all your data: machine learning supercomputers to fight terrorism.
• How IBM's Watson computer used predictive modeling to answer questions and beat the human champs on TV's Jeopardy!
• How companies ascertain untold, private truths — how Target figures out you're pregnant and Hewlett-Packard deduces you're about to quit your job.
• How judges and parole boards rely on crime-predicting computers to decide how long convicts remain in prison.
• 182 examples from Airbnb, the BBC, Citibank, ConEd, Facebook, Ford, Google, the IRS, LinkedIn, Match.com, MTV, Netflix, PayPal, Pfizer, Spotify, Uber, UPS, Wikipedia, and more.
How does predictive analytics work? This jam-packed book satisfies by demystifying the intriguing science under the hood. For future hands-on practitioners pursuing a career in the field, it sets a strong foundation, delivers the prerequisite knowledge, and whets your appetite for more.
A truly omnipresent science, predictive analytics constantly affects our daily lives. Whether you are a consumer of it — or consumed by it — get a handle on the power of Predictive Analytics.
Table of Contents
Foreword
Thomas H. Davenport xiii
Preface to the Revised and Updated Edition
What's new and who's this book for—the Predictive Analytics FAQ
Preface to the Original Edition xv
What is the occupational hazard of predictive analytics?
Introduction
The Prediction Effect 1
How does predicting human behavior combat risk, fortify healthcare, toughen crime fighting, and boost sales? Why must a computer learn in order to predict? How can lousy predictions be extremely valuable? What makes data exceptionally exciting? How is data science like porn? Why shouldn't computers be called computers? Why do organizations predict when you will die?
Chapter 1 Liftoff! Prediction Takes Action (deployment) 17
How much guts does it take to deploy a predictive model into field operation, and what do you stand to gain? What happens when a man invests his entire life savings into his own predictive stock market trading system?
Chapter 2 With Power Comes Responsibility: Hewlett-Packard, Target, the Cops, and the NSA Deduce Your Secrets (ethics) 37
How do we safely harness a predictive machine that can foresee job resignation, pregnancy, and crime? Are civil liberties at risk? Why does one leading health insurance company predict policyholder death? Two extended sidebars reveal: 1) Does the government undertake fraud detection more for its citizens or for self-preservation, and 2) for what compelling purpose does the NSA need your data even if you have no connection to crime whatsoever, and can the agency use machine learning supercomputers to fight terrorism without endangering human rights?
Chapter 3 The Data Effect: A Glut at the End of the Rainbow (data) 67
We are up to our ears in data. How much can this raw material really tell us? What actually makes it predictive? What are the most bizarre discoveries from data? When we find an interesting insight, why are we often better off not asking why? In what way is bigger data more dangerous? How do we avoid being fooled by random noise and ensure scientific discoveries are trustworthy?
Chapter 4 The Machine That Learns: A Look Inside Chase’s Prediction of Mortgage Risk (modeling) 103
What form of risk has the perfect disguise? How does prediction transform risk to opportunity? What should all businesses learn from insurance companies? Why does machine learning require art in addition to science? What kind of predictive model can be understood by everyone? How can we confidently trust a machine's predictions? Why couldn't prediction prevent the global financial crisis?
Chapter 5 The Ensemble Effect: Netflix, Crowdsourcing, and Supercharging Prediction (ensembles) 133
To crowdsource predictive analytics—outsource it to the public at large—a company launches its strategy, data, and research discoveries into the public spotlight. How can this possibly help the company compete? What key innovation in predictive analytics has crowdsourcing helped develop? Must supercharging predictive precision involve overwhelming complexity, or is there an elegant solution? Is there wisdom in nonhuman crowds?
Chapter 6 Watson and the Jeopardy! Challenge (question answering) 151
How does Watson—IBM's Jeopardy!-playing computer—work? Why does it need predictive modeling in order to answer questions, and what secret sauce empowers its high performance? How does the iPhone’s Siri compare? Why is human language such a challenge for computers? Is artificial intelligence possible?
Chapter 7 Persuasion by the Numbers: How Telenor, U.S. Bank, and the Obama Campaign Engineered Influence (uplift) 187
What is the scientific key to persuasion? Why does some marketing fiercely backfire? Why is human behavior the wrong thing to predict? What should all businesses learn about persuasion from presidential campaigns? What voter predictions helped Obama win in 2012 more than the detection of swing voters? How could doctors kill fewer patients inadvertently? How is a person like a quantum particle? Riddle: What often happens to you that cannot be perceived, and that you can't even be sure has happened afterward—but that can be predicted in advance?
Afterword 218
Eleven Predictions for the First Hour of 2022
Appendices
A. The Five Effects of Prediction 221
B. Twenty Applications of Predictive Analytics 222
C. Prediction People—Cast of "Characters" 225
Notes 228
Acknowledgments 290
About the Author 292
Index 293
[hide]
[/hide]
扫码或添加微信号:坛友素质互助
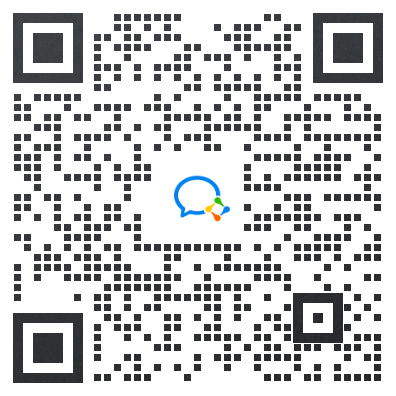
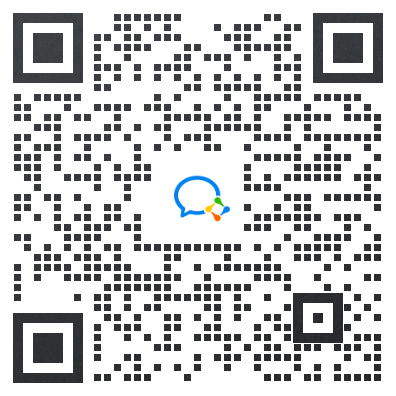
「经管之家」APP:经管人学习、答疑、交友,就上经管之家!
免流量费下载资料----在经管之家app可以下载论坛上的所有资源,并且不额外收取下载高峰期的论坛币。
涵盖所有经管领域的优秀内容----覆盖经济、管理、金融投资、计量统计、数据分析、国贸、财会等专业的学习宝库,各类资料应有尽有。
来自五湖四海的经管达人----已经有上千万的经管人来到这里,你可以找到任何学科方向、有共同话题的朋友。
经管之家(原人大经济论坛),跨越高校的围墙,带你走进经管知识的新世界。
扫描下方二维码下载并注册APP
免流量费下载资料----在经管之家app可以下载论坛上的所有资源,并且不额外收取下载高峰期的论坛币。
涵盖所有经管领域的优秀内容----覆盖经济、管理、金融投资、计量统计、数据分析、国贸、财会等专业的学习宝库,各类资料应有尽有。
来自五湖四海的经管达人----已经有上千万的经管人来到这里,你可以找到任何学科方向、有共同话题的朋友。
经管之家(原人大经济论坛),跨越高校的围墙,带你走进经管知识的新世界。
扫描下方二维码下载并注册APP

您可能感兴趣的文章
本站推荐的文章
人气文章
本文标题:【预测分析,Wiley】Predictive Analytics (2016, 2nd Edition) by Eric Siegel
本文链接网址:https://bbs.pinggu.org/jg/kaoyankaobo_kaoyan_4910576_1.html
2.转载的文章仅代表原创作者观点,与本站无关。其原创性以及文中陈述文字和内容未经本站证实,本站对该文以及其中全部或者部分内容、文字的真实性、完整性、及时性,不作出任何保证或承若;
3.如本站转载稿涉及版权等问题,请作者及时联系本站,我们会及时处理。