关于本站
人大经济论坛-经管之家:分享大学、考研、论文、会计、留学、数据、经济学、金融学、管理学、统计学、博弈论、统计年鉴、行业分析包括等相关资源。
经管之家是国内活跃的在线教育咨询平台!
经管之家新媒体交易平台
提供"微信号、微博、抖音、快手、头条、小红书、百家号、企鹅号、UC号、一点资讯"等虚拟账号交易,真正实现买卖双方的共赢。【请点击这里访问】
论文
- 毕业论文 | 写毕业论文
- 毕业论文 | 为毕业论文找思路
- 毕业论文 | 可以有时间好好写 ...
- 毕业论文 | 毕业论文如何选较 ...
- 毕业论文 | 毕业论文选题通过 ...
- 毕业论文 | 还有三人的毕业论 ...
- 毕业论文 | 毕业论文答辩过程 ...
- 毕业论文 | 本科毕业论文,wi ...
考研考博
- 考博 | 南大考博经济类资 ...
- 考博 | 考博英语10000词汇 ...
- 考博 | 如果复旦、南大这 ...
- 考博 | 有谁知道春招秋季 ...
- 考博 | 工作与考博?到底 ...
- 考博 | 考博应该如何选择 ...
- 考博 | 考博失败了
- 考博 | 考博考研英语作文 ...
留学
- 日本留学 | 在日本留学心得
- 日本留学 | 日本留学生活必需 ...
- 日本留学 | 【留学日本】2015 ...
- 日本留学 | 日本海外留学8年来 ...
- 日本留学 | 日本留学费用_日本 ...
- 日本留学 | 求在日本留学的师 ...
- 日本留学 | 日本留学的有没有 ...
- 日本留学 | 日本留学
TOP热门关键词
RegressionAndMediationAnalysisUsingMpluspdf下载TheinspirationtowritethisbookcamefrommanyyearsofteachingaboutMplusandansweringquestionsonMplusDiscussionandMplussupport.Itbecameclearthatoncepeopleleaves ...
坛友互助群![]() |
扫码加入各岗位、行业、专业交流群![]() |
The inspiration to write this book came from many years of teaching about Mplus and answering questions on Mplus Discussion and Mplus support. It became clear that once people leave school, it is difficult to keep up with the newest methodology. The purpose of this book is to provide researchers with information that is not readily available to them and that we believe is important for their research. Many topics such as linear regression analysis; mediation analysis; causal inference; regression analysis with categorical, count, and censored outcomes; Bayesian analysis; and missing data analysis have entire books devoted to them. This book does not attempt to replace these books but rather to give useful and manageable summaries of these topics and show how the analyses are implemented in Mplus. The technical level is kept at a minimum but still requires an introductory statistics background and a good background in regression.
Chapter 1 covers linear regression analysis including regression with an interaction, multiple-group analysis, missing data on covariates, and heteroscedasticity modeling. Chapter 2 covers mediation analysis with a continuous mediator and a continuous outcome including moderated mediation. Chapter 3 covers special topics in mediation analysis that are not normally found in books on mediation analysis. These include Monte Carlo simulation studies of mediation and moderated mediation, model misspecification due to omitted variables and confounders, instrumental variable estimation, sensitivity analysis, multiple group analysis of moderated mediation, and measurement error. Chapter 4 covers causal inference based on counterfactuals for mediation analysis with a continuous mediator and a continuous outcome. Chapter 5 covers regression analysis for categorical dependent variables including binary, ordinal, and nominal variables. Chapter 6 covers regression analysis for a count dependent variable including the following models: Poisson, Poisson with a random intercept, zero-inflated Poisson, negative binomial, zero-inflated negative binomial, two-part (hurdle) with zero-truncation, and varying-exposure. Chapter 7 covers regression analysis for a censored dependent variable including the following models: censored-normal (tobit), censored-inflated, sample selection (Heckman), two-part, and switching regressions. Chapter 8 covers causal inference for mediation analysis with a binary outcome and a continuous mediator, a count outcome and a continuous mediator, a two- part outcome and a continuous mediator, a binary and an ordinal mediator, a nominal mediator, and a mediator with measurement error. Chapter 9 discusses Bayesian analysis and uses it to estimate several mediation examples which show how it can be used as an alternative to maximum likelihood estimation. Chapter 10 discusses several approaches to missing data modelling including missing completely at random (MCAR), missing at random (MAR), and not missing at random (NMAR) including selection modeling.
Table of Contents
Preface
Linear regression analysis
Linear regression analysis assumptions
Linear regression estimation
Residuals
Outliers
Linear regression R-square
Linear regression standardization
Example: Regression with one covariate
Individual residuals and outliers
Reporting results
Multiple covariates
Linear regression with two continuous covariates
Interaction between two continuous covariates
Linear regression with one binary and one continuous covariate
Interaction between a binary and a continuous covariate
Example: Regression with two covariates
Reporting results
Example: Regression with an interaction
Reporting results
Presenting parameter estimates
Presenting results graphically
Special topics
Standardized coefficients greater than one
Standardized coefficients differing in significance from unstandardized coefficients
Two-group regression analysis
Example: Two-group regression analysis of an intervention study
Bringing covariates into the model
Missing data on x
Example: Bringing a covariate into the model for the intervention example using a two-group analysis
The Bayesian Information Criterion (BIC) and the Akaike Information Criterion (AIC)
Heteroscedasticity modeling
Example: Heteroscedasticity modeling of LSAY math data
Random coefficient regression
Example: Random coefficient regression for LSAY math data
Mediation analysis
A prototypical mediation model
Mediation modeling techniques
Estimation
Indirect effect standard errors and confidence intervals
Standardization for mediation models
Model testing
Example: Sex discrimination
Inspecting the data and reporting results
Example: Head circumference
Reporting results
The saturated model
Multiple mediators
Example: Parallel mediators for media influence
Example: Sequential mediators of socioeconomic status
Moderated mediation
Case 1 (xz): Regression of y on x, m on x, both moderated by z
Case 2 (mz): Regression of y on m moderated by z
Case 3 (mx): Regression of y on m moderated by x
Combined moderation case
Example: Case 1 moderated mediation in an intervention of aggressive behavior in the classroom
Testing significance of effects at specific moderator values
Creating a plot with bootstrap confidence intervals for the effects at a range of moderator values
Combination of significance of effect at specific moderator values and plot of confidence intervals using MODEL INDIRECT
Example: Case 2 moderated mediation for work team behavior
Example: Case 3 moderated mediation of simulated data
Example: Combined moderated mediation for sex discrimination
Special topics in mediation analysis
Monte Carlo simulation study of mediation
Example: Monte Carlo study of indirect effects
Monte Carlo studies of moderation
Example: Moderation of the regression of m on x
Example: Moderation of the regression of y on m
Model misspecification
Example: Omitted moderator
Example: Omitted mediators
Example: Confounders
Instrumental variable estimation
Example: IV estimation with mediator-outcome confounding
Bias and coverage of IV estimation of the indirect effect
Comparing IV and ML standard errors and power
IV estimation dependence on the size of the x, m correlation
Comparison of IV and maximum-likelihood estima- tion when the assumptions behind both approaches are violated
Sensitivity analysis
Example: Sensitivity analysis for an experimental study of sex discrimination in the workplace
Example: Sensitivity analysis in a Monte Carlo study
Multiple-group mediation analysis
Relating multiple-group parameters to interaction parameters
Modification indices
Example: Two-group analysis of moderated mediation for sex discrimination
Measurement errors and latent variables
Measurement error in an independent variable
Measurement error in a mediator
Example: Monte Carlo simulation study of measurement error in the mediator
Known reliability
Multiple indicators
Reliability of a sum of indicators
Structural equation modeling with a factor analysis measurement model
Causal inference for mediation
Causal assumptions
Potential outcomes and counterfactuals
Example: Hypothetical potential outcome data
Basics of counterfactually-defined effects
Example: Hypothetical mediation potential outcomes
Direct and indirect effects
Direct effects
Indirect effects
Total effect decomposition
Example: Hypothetical mediation data analysis
Causal effect formulas
Example: Effects in the simple mediation case
Example: Effects with moderation of Y regressed on M (case 3)
Example: Effects in the combined moderation case
Example: Effects combining case 1 and case 2 moderation
Multiple mediators
Summary
Categorical dependent variable
Basic concepts for categorical variables
Binary variables
Binary dependent variable
Example: OLS, logistic, and probit regression of coal miner respiratory problems
Modeling with a logistic regression function
Modeling with a probit regression function
Estimation of the logistic and probit regressions
Probability curve formulation versus a latent response variable formulation
R2 and standardization
R2 for a binary outcome
Standardization
Example: Logistic and probit regression of British coal miner data
Logistic regression
Probit regression
Computation of estimated probabilities
Comparing logistic and probit regression coefficients
Comparing the logistic and probit regression models by BIC
Logistic and probit regression with one binary and one continuous x
Logistic regression and adjusted odds ratios
Example: Adjusted odds ratios for alcohol survey data
Example: Adjusted odds ratios for educational achievement data
Ordinal dependent variable
Probability curve formulation of ordinal dependent variable regression
Latent response variable formulation of ordinal dependent variable regression
Example: Sample probits for drinking related to age and income
Testing the parallel probability curve assumption behind the ordinal regression model
Example: Ordinal logistic regression of mental impairment
Estimated odds ratio
Estimated probabilities
Odds ratio with an interaction
Nominal dependent variable
Example: Multinomial logistic regression of antisocial behavior
Count dependent variable
Poisson model
Poisson model with a random intercept
Zero-inflated Poisson model
Negative binomial model
Zero-inflated negative binomial model
Two-part (hurdle) model with zero-truncation
Varying-exposure model
Comparing models
Example: Count regression of marital affairs
Poisson, Poisson with a random intercept, and negative binomial models
Negative binomial model
Zero-inflated Poisson and zero-inflated negative binomial models
Two-part (hurdle) modeling
Conclusion for marital affairs analyses
Example: Poisson with varying exposure
Censored dependent variable
Basic concepts for a censored variable
Censored-normal (tobit) regression
Censored-inflated regression
Sample selection (Heckman) regression
Example: Simulated sample selection data
Two-part regression
Example: Methods comparison on alcohol data
Analysis results for the four models
Loglikelihood and BIC comparisons of the four models
Comparing the results for the censored-normal (tobit) and censored-inflated models
Comparing the results for the sample selection (Heckman) and two-part models
Comparing the results for the censored-inflated and two-part models
Comparing the fit for estimated probabilities and means for the censored-inflated and two-part models
Switching regressions
Example: Monte Carlo simulation of switching regressions
Mediation non-continuous variables
Binary outcome, continuous mediator
A simple hypothetical mediation model
Total, indirect, and direct effects in terms of differences in probabilities
Causal effect formulas for a continuous M and a binary Y
Causal effect formulas applied to a simple mediation model with a binary outcome and a continuous mediator
Causal effect formulas defined on the odds ratio scale
Odds ratio effects assuming a rare outcome
Example: Intention to use cigarettes
Probit regression
Logistic regression
Example: HPV vaccination trial
No intervention-mediator interaction
Intervention-mediator interaction
Analysis results
Count outcome, continuous mediator
Causal effect formulas for a count outcome
Example: Aggressive behavior and school removal
Estimated count probabilities
Two-part outcome, continuous mediator
Causal effect formulas for a two-part outcome
Example: Two-part mediation analysis of economic stress data
Causal effects for two-part modeling
Causal effects for regular modeling with log y
Causal effects for regular modeling without log y
Binary and ordinal mediator
Causal effect formulas for a binary mediator
Ordinal mediator
Latent response variable mediator
Estimation
Example: Hypothetical data from the potential outcome example with a binary mediator and a continuous outcome
Example: Ordinal mediator for intention to use cigarettes
Example: Pearl’s artificial 2 x 2 x 2 example
Nominal mediator
Causal effect formulas for a nominal mediator
Estimation
Example: Hypothetical data with a nominal mediator and a binary outcome
Mediator with measurement error
Example: A Monte Carlo simulation study for a mediator measured with error
Example: An intervention study of aggressive behavior in the classroom and juvenile court record
Bayesian analysis
Prior, likelihood, and posterior
Posterior distribution for the mean of a normal distribution
Types of priors
Non-normality of parameter distributions
Markov Chain Monte Carlo (MCMC)
Example: Bayesian estimation of a mean with missing data for LSAY math
Input for Bayesian analysis
Plots
Trace plot
Autocorrelation plot
Posterior distribution plot
Convergence checking
Model fit
Bayes versus ML intervals
Example: Mediation model for media influence
The Potential Scale Reduction (PSR) convergence criterion
Trace plot
Autocorrelation plot
Inspecting model fit and parameter estimates
Example: Mediation model for firefighter data
Non-informative priors
Informative priors
Example: Model testing of direct effects
Example: High school dropout and missing data
Missing data on the mediator (n = 2, 213)
Bayesian analysis
Maximum-likelihood analysis
Missing data on the control variables (n = 2, 898)
Assuming normality for all covariates
Acknowledging that some control variables are binary
Missing data
Example: Missing data information
MCAR, MAR, and NMAR
MCAR: Missing completely at random
MAR: Missing at random
NMAR: Not missing at random
MAR for bivariate normal variables (H1 case)
Listwise versus ML
Maximum-likelihood estimation in the bivariate case with missing on one variable
The EM algorithm
Multiple imputation
Example: Estimating sample statistics for intervention data
MAR for regression (H0 case)
Missing data and selection on x or y
Regression analysis with missing data
Technical aspects of ML assuming MAR
Example: MAR simulated data analysis
Missing data correlates
Example: Simulation study with missing data correlate of missing on y
NMAR
Example: Simulated NMAR data with missing influenced by the latent outcome
Example: Selection modeling versus ML assuming MAR when MAR holds
Example: Comparing missing data methods
Missing data on covariates
Example: Simulation study of missing on binary covariates
Inputs for generating and analyzing data assuming MAR
Inputs for generating and analyzing data under NMAR
Simulation results
扫码或添加微信号:坛友素质互助
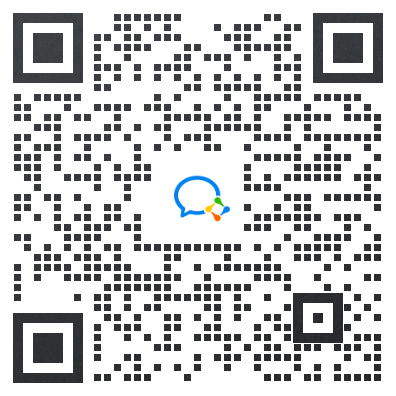
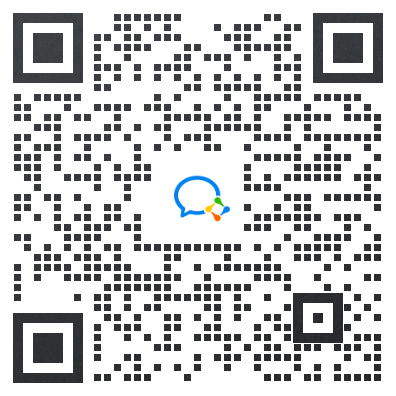
「经管之家」APP:经管人学习、答疑、交友,就上经管之家!
免流量费下载资料----在经管之家app可以下载论坛上的所有资源,并且不额外收取下载高峰期的论坛币。
涵盖所有经管领域的优秀内容----覆盖经济、管理、金融投资、计量统计、数据分析、国贸、财会等专业的学习宝库,各类资料应有尽有。
来自五湖四海的经管达人----已经有上千万的经管人来到这里,你可以找到任何学科方向、有共同话题的朋友。
经管之家(原人大经济论坛),跨越高校的围墙,带你走进经管知识的新世界。
扫描下方二维码下载并注册APP
免流量费下载资料----在经管之家app可以下载论坛上的所有资源,并且不额外收取下载高峰期的论坛币。
涵盖所有经管领域的优秀内容----覆盖经济、管理、金融投资、计量统计、数据分析、国贸、财会等专业的学习宝库,各类资料应有尽有。
来自五湖四海的经管达人----已经有上千万的经管人来到这里,你可以找到任何学科方向、有共同话题的朋友。
经管之家(原人大经济论坛),跨越高校的围墙,带你走进经管知识的新世界。
扫描下方二维码下载并注册APP

您可能感兴趣的文章
本站推荐的文章
- 哲学名言 | 【独家发布】经典哲学名言
- 哲学书籍 | 求推荐一本讲人生目标的哲学书籍 ...
- 哲学书籍 | 20部必读的哲学书籍
- 哲学书籍 | 经济人,开拓你逻辑思维的哲学书 ...
- 哲学书籍 | 哲学书籍
- 哲学书籍 | 哲学书籍
- 哲学书籍 | 哲学书籍
- 哲学书籍 | 经典的哲学书籍
人气文章
本文标题:Regression And Mediation Analysis Using Mplus pdf下载
本文链接网址:https://bbs.pinggu.org/jg/huiji_huijiku_4889172_1.html
2.转载的文章仅代表原创作者观点,与本站无关。其原创性以及文中陈述文字和内容未经本站证实,本站对该文以及其中全部或者部分内容、文字的真实性、完整性、及时性,不作出任何保证或承若;
3.如本站转载稿涉及版权等问题,请作者及时联系本站,我们会及时处理。