Dummy Coding Nominal Variables
Dichotomously coded nominal variables can always be used in a linear model (as in multiple regression and discriminant analysis). However, variables that have more than two levels, can not be used as coded as predictors in such models. Thus, gender coded, for instance, as 0=male and 1=female, presents no problem nor does political party coded as 1=democrat and 2=republican. But if we wished to have three or more political parties, the variable coded as 1=democrat, 2=republican, and 3=independent could not be used. If we did use political party coded in this way, SPSS would happily do our analysis, but the answer would be meaningless as our coding implies that republican is "more" than democrat and that independent is "more" than republican or democrat. We imply at least an ordinal scale by our coding, which, as political party is nominal, is incorrect.
If the variable IS ordinal, such as class standing (Freshman, Sophomore, Junior, Senior), you can use it coded ordinally (e.g.., 1, 2, 3, 4), and the method discussed herein should NOT be applied.
As we can use dichotomous variables, what we must do is to transform our polychotomous nominal variable into a set of dichotomous, or binary, variables. Fortunately, we can always transform a k-level nominal variable into a set of k-1 binary variables that contain the same information. Thus in this case, we can transform the 3-level nominal political party variable into 2 binary variables, and then use the two binary variables as the representation for political party in any modeling procedure, such as multiple regression, discriminant analysis, etc.
There is more than one way to create these binary variables; the procedure illustrated herein is often referred to as "dummy coding." What we do is to create k-1 variables each representing, by "0" or "1", whether the subject is a member of each of k-1 groups, thus we elect to not represent this information for one of the groups as to do so would be redundant. For our political party example, let's say that we have a variable PARTY,coded as 1, 2, or 3 as prescribed above. It is arbitrary which party we "leave out" in creating k-1 binary variables, but for this example, lets code whether the subject is a democrat, "D," and whether the subject is a republican, R. The point is that if there is a "0" for both of these, then the subject is a member of the remaining Independent group. That is why we do not need to code k binary variables -- only k-1. The coding for Ss of all types would look something like this:
S# Party D R
1 1 1 0
2 2 0 1
3 3 0 0
Although this represents the coding for a subject of each type, all subjects would be coded similarly for PARTY.
Although we could manually create the dummy coded variables, we will have SPSS create the dummy coded variables for us. We assume that the nominal variable, as in PARTY, is already extant in the file as in the example below (the file, DUMMY.sav, is included in this folder):
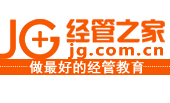
You will note that we also have the variable age in this example. I included this so that I can show you how the dummy codes can be used, and an additional statistical concept, but it has nothing to do with dummy coding.
Now, to get the required two dummy coded variables for the three category nominal variable, PARTY, we use the SPSS "Recode into Different Variables" command.
What we need to do is to create two binary dummy coded variables, each representing membership or not in two of the three levels of PARTY. We choose to represent membership in the democratic party or not with the dummy coded variable D, and the same for the republican party with R. In order to accomplish this we click "Transform/Recode/Into Different Variables..." This opens a dialog box. We move the variable PARTY to the Input Variable box, and type D in the Output Variable box. The window should look something like this:
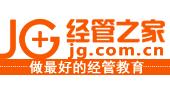
Next, click the Old and New Values button, and another dialog box opens. Enter the value "1" in the Old Value box (this represents the value of PARTY for Democrat), and "1" in the New Value box. Then click theAdd button. The transformation of that value (although it is from the same value to the same value) appears in the window recording such changes to the left of the dialog box. Next click the All other values selection on the left, "0" on the right, and the Add button. This causes the rest of the values of PARTY (ELSE) to be transformed to "0" in the new variable D. Thus these two transformations create a new variable, D, that is "1" if the subject is a democrat and "0" otherwise, just as we wished. The screen should look something like this:
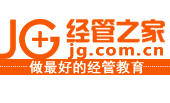
Next click the Continue button. You will go back to the former "Recode into Different Variables" window. You must click the Change button for the changes to take effect. You could also enter a Label for the variableD if you wish. Click OK and the new variable, D, will be created and added to the end of the data file. You can do the same thing to create the R dummy coded variable, except that the transformation consists of 2 > 1, and ELSE > 0, as it is to be a binary variable registering whether the subject is a republican or not. Before creating the R variable, you will need to "clear out" the "party > D" entry in the "Recode into Different Variables" dialog box (as it will still be there from creating the D variable) by highlighting it and clicking the left arrow. You will also need to clear the coding selections that you made for D in the "Old and New Values" dialog box by highlighting and clicking the Remove button. I leave that exercise for you. You can also enter Variable Values for D and R if you wish. I did so in the DUMMY.sav file. They were "democrat" and "not democrat" and similarly for R.
After creating both dummy coded variables the file looks like this:
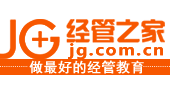
Here you can see that we have the two desired new variables, D and R, and that a democrat is coded 1, 0, a republican is 0, 1, and an independent is 0, 0; thus, we have all of the information that was in the nominal variable, PARTY.
The k-1 dummy codes that represent the k-level nominal variable (but not the original nominal variable) would now be added into any linear modeling technique (such as multiple regression or discriminant analysis) together, and they together represent the predictive power of the nominal variable. Though you will typically have many variables in a model, some of which are dummy coded and some which are continuous variables, I illustrate using just these dummy coded variables in predicting age, thus we are considering how well we can predict age from political party.
To illustrate how we would use these two dummy coded variables together to represent PARTY, let's run a regression predicting age from D and R. We would set up the regression as usual, including D and R as Independents and age as the Dependent variable (note that PARTY is not included in the analysis). The relevant window looks like this:
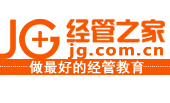
The basic output is:
ANOVA
Model | | Sum of Squares | df | Mean Square | F | Sig. |
1 | Regression | 79.000 | 2 | 39.500 | .562 | .621(a) |
Residual | 211.000 | 3 | 70.333 | | |
Total | 290.000 | 5 | | | |
a Predictors: (Constant), R, D
b Dependent Variable: AGE
As an alternative, let's set up the ONEWAY ANOVA procedure, testing the null hypothesis that the means of the political parties are the same (here we can use PARTY in its original state, and we have no use for D andR) as ANOVA expects a nominal independent variable . The window looks like this:
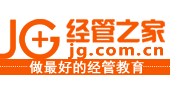
The output from this ANOVA is:
ANOVA
AGE
| Sum of Squares | df | Mean Square | F | Sig. |
Between Groups | 79.000 | 2 | 39.500 | .562 | .621 |
Within Groups | 211.000 | 3 | 70.333 | | |
Total | 290.000 | 5 | | | |
We see that the result is the same whether we are asking the question of how well we can predict age from political party, or is there a difference in mean age across political parties -- the question is the same statistically.
One would not go through the trouble of recoding the PARTY variable if it were the only variable of interest, as in this example; we could just do an ANOVA. However, when there are multiple variables, some of which are nominal and some of which are not, we can dummy code those that are nominal and use the dummy coded variables to represent the nominal variable in a model utilizing all predictors.